Machine Learning: Are You Ready To Succeed?
- 4
- minutes to read
- minutes to read
In today’s highly digitised marketing landscape, the words ‘machine learning’ and ‘artificial intelligence’ are appearing more frequently than ever. Many companies are struggling to understand whether they should invest in machine learning to set themselves up for future success or how it can be leveraged to improve current marketing efforts.
This is extremely difficult as most people have a limited view of what machine learning involves and fundamentally what exactly these practices can enable.
This article will explain some of the key considerations you can use to determine if you are ready to embark on implementing machine learning practices and highlight some key differences between analytics, machine learning and artificial intelligence.
Navigating the intertwined relationship of analytics and machine learning
Machine learning is often confused with artificial intelligence and analytics, so let’s be clear on how they differ and what they have in common.
Predictive analytics and machine learning as a process are essentially the same thing, they both:
- Take your clean data set and make it available to the analytics team or machine learning model (or your unclean data, more on this later).
- Mine your data for insights and opportunities.
- Visualise your findings for the masses to understand.
- Take the learnt changes about your trends or customer behaviour, apply these and repeat the process with your new knowledge.
Where predictive analytics and machine learning differ is in the human involvement from points 2–4. In predictive analytics this would all be completed by an analyst or team of analysts within your organisation. However, machine learning allows you to define how you would like the data to be analysed and essentially automate this task after the initial build.
When someone reads an article or blog outlining machine learning, usually their immediate thought is, “wait…can machine learning remove my need for manual analytics?” The short answer is no.
The better answer is that machine learning should be considered as an extension of your analytics process. It should be an extremely useful tool in speeding up processes and reducing the amount of manual work. This will free up time for your analytics function to focus on tasks that machine learning cannot currently solve and ensure that the machine learning process is regularly checked and improved.
Artificial intelligence (AI) is a further extension of analytics, where machine learning takes a defined rule set and conducts analysis to seek out common patterns. AI focuses on how it can take what it has learnt and apply it to new scenarios, essentially taking a more ‘human approach’ to finding answers.
Key considerations before implementing machine learning
Implementing a new process or function is often daunting and it is also daunting for good reason. Without proper planning and investment from the wider business these projects often fail or don’t deliver the true value that was expected before it was commenced.
It doesn’t have to be this way! Ensuring you are ready to implement machine learning is the first step to make certain the process runs smoothly. There are plenty of examples where companies got it right. You can follow these examples to guarantee you are successful in leveraging this process to meet your goals.
Here is a list of key steps that appear time and time again in scenarios where a company got it right:
Define your machine learning goal and use cases first to ensure these are aligned with your wider marketing and company goals.
Clean your data. Like any analytics process or project, your output is only as good as your input — later in this piece we dig deeper into this and provide some practical steps as to how you should go about this.
Leverage your analysts. Bring them in early and confirm that they are invested in the project. There is a worldwide shortage of trained data scientists. Upskill your analysts that already have a profound understanding of your business and data…this will always be a sounds investment.
Start small and learn fast. Taking an iterative approach to machine learning allows for smaller initial investment. Starting small also provides valuable results from initial models that you can then scale efficiently and effectively. Remember to value your failures, they are the key to moving forward faster.
Defining your ‘clean’ strategy
Your data strategy needs to consider your objective and how each of your data points will help push you towards that goal. If your data strategy is not tied to the overall business objectives, it will only show you what is available but not ‘how’ to use it. Having mountains of data is unnecessary; you just need complete, accurate and timely data that services your goals.
In many ways ‘tech’ is the easy part — ensuring your data is clean, structured, and accessible is where it gets tricky. Getting rid of dirty data and thoroughly cleaning it up is the key to producing a machine learning practice that is both scalable and flexible. A good data strategy always looks beyond ‘keeping up’ or ‘staying ahead’ of competitors. It aims to exceed customers’ expectations by proactively understanding their needs first.
What does success look like?
A strong data strategy will assist in surfacing the necessary information, allowing you to solve both relevancy and helpfulness. Meanwhile the function of machine learning, whether it is people, process, or technology, will assist with speed and cost factors. In a perfect world all these functions work together seamlessly. This relieves time otherwise spent on manual processes to be redirected into improving data strategy and machine learning practices.
If you have any questions, please reach out to hello@thelumery.com
Written by
- –
- Senior Strategist
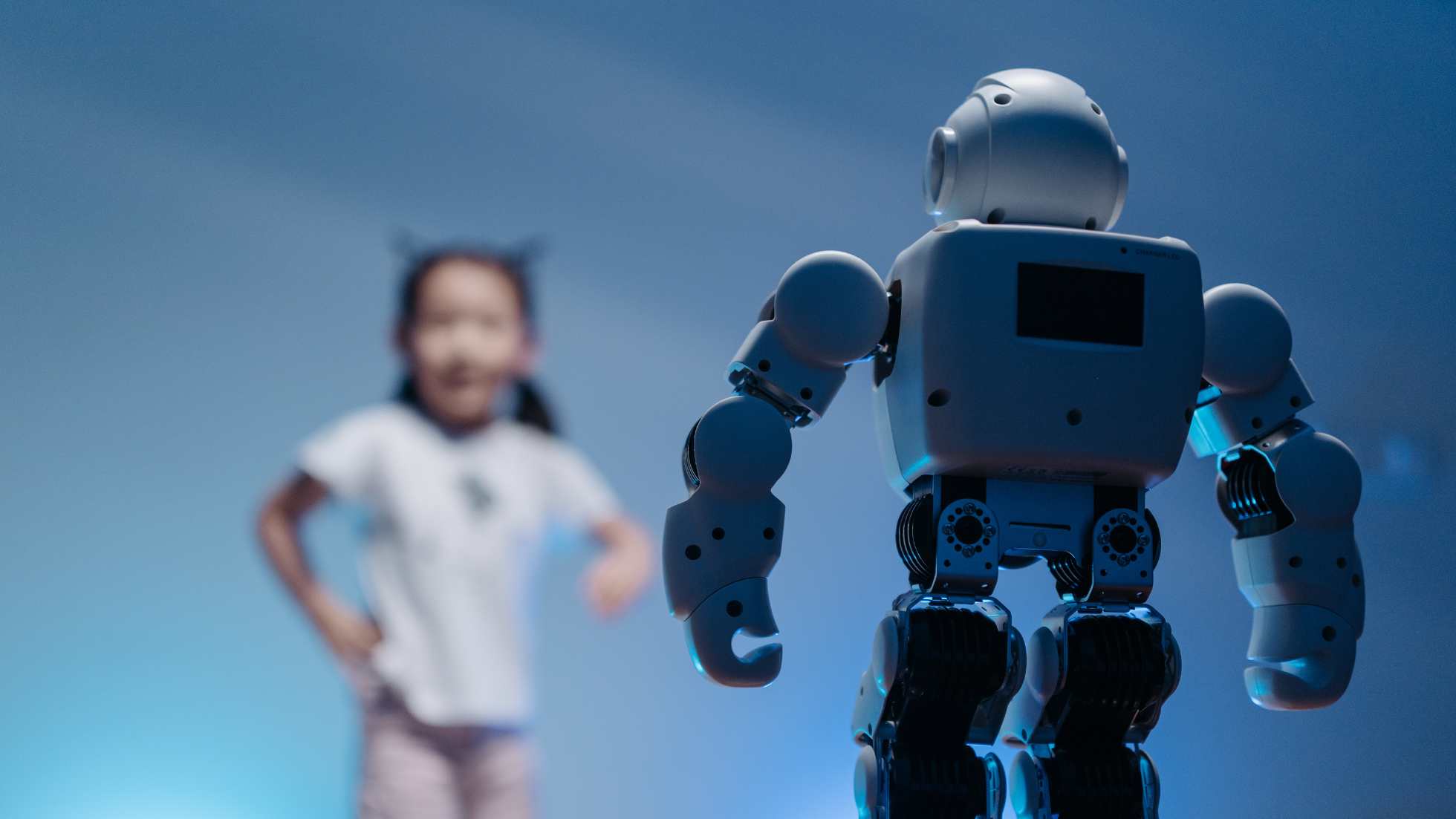